Conv2D 내부 구조 및 시간복잡도
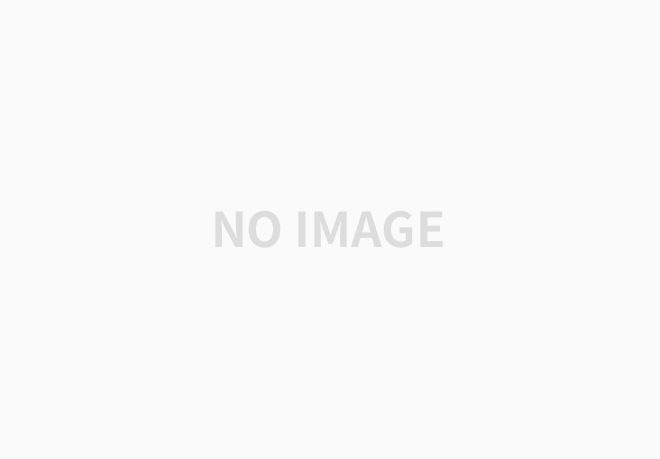
지난 1편 때,
Conv2D에 대한 개념적인 설명은 충분히 했지만,
이미지 분류의 주류를 이루는, 그리고 그것 중 핵심이라고 할 수 있는 Convolution Layer를 설명하면서,
실제 내부 구조가 어떻게 이루어져 있는지에 대한 설명은 빠진 것 같았다.
그래서 이번에는 매우 복잡하지만,그 내부가 어떻게 이루어져 있는지 알아볼 예정이다.
Tensorflow 2.X
# Tensorflow 2.X Conv2D
# https://github.com/tensorflow/tensorflow/blob/master/tensorflow/python/keras/layers/convolutional.py
@keras_export('keras.layers.Conv2D', 'keras.layers.Convolution2D')
class Conv2D(Conv):
def __init__(self,
filters,
kernel_size,
strides=(1, 1),
padding='valid',
data_format=None,
dilation_rate=(1, 1),
groups=1,
activation=None,
use_bias=True,
kernel_initializer='glorot_uniform',
bias_initializer='zeros',
kernel_regularizer=None,
bias_regularizer=None,
activity_regularizer=None,
kernel_constraint=None,
bias_constraint=None,
**kwargs):
super(Conv2D, self).__init__(
rank=2,
filters=filters,
kernel_size=kernel_size,
strides=strides,
padding=padding,
data_format=data_format,
dilation_rate=dilation_rate,
groups=groups,
activation=activations.get(activation),
use_bias=use_bias,
kernel_initializer=initializers.get(kernel_initializer),
bias_initializer=initializers.get(bias_initializer),
kernel_regularizer=regularizers.get(kernel_regularizer),
bias_regularizer=regularizers.get(bias_regularizer),
activity_regularizer=regularizers.get(activity_regularizer),
kernel_constraint=constraints.get(kernel_constraint),
bias_constraint=constraints.get(bias_constraint),
**kwargs)
Tensorflow Official Github에서 convolutional.py 문서에서 가져온 Conv2D 클래스이다.
보다시피 Conv2D 클래스는 Conv 클래스를 상속 받았으므로 Conv 클래스를 한 번 보도록 하자.
# Tensorflow 2.X Conv Class
# https://github.com/tensorflow/tensorflow/blob/master/tensorflow/python/keras/layers/convolutional.py
class Conv(Layer):
def __init__(self,
rank,
filters,
kernel_size,
strides=1,
padding='valid',
data_format=None,
dilation_rate=1,
groups=1,
activation=None,
use_bias=True,
kernel_initializer='glorot_uniform',
bias_initializer='zeros',
kernel_regularizer=None,
bias_regularizer=None,
activity_regularizer=None,
kernel_constraint=None,
bias_constraint=None,
trainable=True,
name=None,
conv_op=None,
**kwargs):
super(Conv, self).__init__(
trainable=trainable,
name=name,
activity_regularizer=regularizers.get(activity_regularizer),
**kwargs)
self.rank = rank
if isinstance(filters, float):
filters = int(filters)
self.filters = filters
self.groups = groups or 1
self.kernel_size = conv_utils.normalize_tuple(
kernel_size, rank, 'kernel_size')
self.strides = conv_utils.normalize_tuple(strides, rank, 'strides')
self.padding = conv_utils.normalize_padding(padding)
self.data_format = conv_utils.normalize_data_format(data_format)
self.dilation_rate = conv_utils.normalize_tuple(
dilation_rate, rank, 'dilation_rate')
self.activation = activations.get(activation)
self.use_bias = use_bias
self.kernel_initializer = initializers.get(kernel_initializer)
self.bias_initializer = initializers.get(bias_initializer)
self.kernel_regularizer = regularizers.get(kernel_regularizer)
self.bias_regularizer = regularizers.get(bias_regularizer)
self.kernel_constraint = constraints.get(kernel_constraint)
self.bias_constraint = constraints.get(bias_constraint)
self.input_spec = InputSpec(min_ndim=self.rank + 2)
self._validate_init()
self._is_causal = self.padding == 'causal'
self._channels_first = self.data_format == 'channels_first'
self._tf_data_format = conv_utils.convert_data_format(
self.data_format, self.rank + 2)
def _validate_init(self):
if self.filters is not None and self.filters % self.groups != 0:
raise ValueError(
'The number of filters must be evenly divisible by the number of '
'groups. Received: groups={}, filters={}'.format(
self.groups, self.filters))
if not all(self.kernel_size):
raise ValueError('The argument `kernel_size` cannot contain 0(s). '
'Received: %s' % (self.kernel_size,))
if (self.padding == 'causal' and not isinstance(self,
(Conv1D, SeparableConv1D))):
raise ValueError('Causal padding is only supported for `Conv1D`'
'and `SeparableConv1D`.')
def build(self, input_shape):
input_shape = tensor_shape.TensorShape(input_shape)
input_channel = self._get_input_channel(input_shape)
if input_channel % self.groups != 0:
raise ValueError(
'The number of input channels must be evenly divisible by the number '
'of groups. Received groups={}, but the input has {} channels '
'(full input shape is {}).'.format(self.groups, input_channel,
input_shape))
kernel_shape = self.kernel_size + (input_channel // self.groups,
self.filters)
self.kernel = self.add_weight(
name='kernel',
shape=kernel_shape,
initializer=self.kernel_initializer,
regularizer=self.kernel_regularizer,
constraint=self.kernel_constraint,
trainable=True,
dtype=self.dtype)
if self.use_bias:
self.bias = self.add_weight(
name='bias',
shape=(self.filters,),
initializer=self.bias_initializer,
regularizer=self.bias_regularizer,
constraint=self.bias_constraint,
trainable=True,
dtype=self.dtype)
else:
self.bias = None
channel_axis = self._get_channel_axis()
self.input_spec = InputSpec(min_ndim=self.rank + 2,
axes={channel_axis: input_channel})
# Convert Keras formats to TF native formats.
if self.padding == 'causal':
tf_padding = 'VALID' # Causal padding handled in `call`.
elif isinstance(self.padding, six.string_types):
tf_padding = self.padding.upper()
else:
tf_padding = self.padding
tf_dilations = list(self.dilation_rate)
tf_strides = list(self.strides)
tf_op_name = self.__class__.__name__
if tf_op_name == 'Conv1D':
tf_op_name = 'conv1d' # Backwards compat.
self._convolution_op = functools.partial(
nn_ops.convolution_v2,
strides=tf_strides,
padding=tf_padding,
dilations=tf_dilations,
data_format=self._tf_data_format,
name=tf_op_name)
self.built = True
def call(self, inputs):
if self._is_causal: # Apply causal padding to inputs for Conv1D.
inputs = array_ops.pad(inputs, self._compute_causal_padding(inputs))
outputs = self._convolution_op(inputs, self.kernel)
if self.use_bias:
output_rank = outputs.shape.rank
if self.rank == 1 and self._channels_first:
# nn.bias_add does not accept a 1D input tensor.
bias = array_ops.reshape(self.bias, (1, self.filters, 1))
outputs += bias
else:
# Handle multiple batch dimensions.
if output_rank is not None and output_rank > 2 + self.rank:
def _apply_fn(o):
return nn.bias_add(o, self.bias, data_format=self._tf_data_format)
outputs = nn_ops.squeeze_batch_dims(
outputs, _apply_fn, inner_rank=self.rank + 1)
else:
outputs = nn.bias_add(
outputs, self.bias, data_format=self._tf_data_format)
if self.activation is not None:
return self.activation(outputs)
return outputs
def _spatial_output_shape(self, spatial_input_shape):
return [
conv_utils.conv_output_length(
length,
self.kernel_size[i],
padding=self.padding,
stride=self.strides[i],
dilation=self.dilation_rate[i])
for i, length in enumerate(spatial_input_shape)
]
def compute_output_shape(self, input_shape):
input_shape = tensor_shape.TensorShape(input_shape).as_list()
batch_rank = len(input_shape) - self.rank - 1
if self.data_format == 'channels_last':
return tensor_shape.TensorShape(
input_shape[:batch_rank]
+ self._spatial_output_shape(input_shape[batch_rank:-1])
+ [self.filters])
else:
return tensor_shape.TensorShape(
input_shape[:batch_rank] + [self.filters] +
self._spatial_output_shape(input_shape[batch_rank + 1:]))
def _recreate_conv_op(self, inputs): # pylint: disable=unused-argument
return False
def get_config(self):
config = {
'filters':
self.filters,
'kernel_size':
self.kernel_size,
'strides':
self.strides,
'padding':
self.padding,
'data_format':
self.data_format,
'dilation_rate':
self.dilation_rate,
'groups':
self.groups,
'activation':
activations.serialize(self.activation),
'use_bias':
self.use_bias,
'kernel_initializer':
initializers.serialize(self.kernel_initializer),
'bias_initializer':
initializers.serialize(self.bias_initializer),
'kernel_regularizer':
regularizers.serialize(self.kernel_regularizer),
'bias_regularizer':
regularizers.serialize(self.bias_regularizer),
'activity_regularizer':
regularizers.serialize(self.activity_regularizer),
'kernel_constraint':
constraints.serialize(self.kernel_constraint),
'bias_constraint':
constraints.serialize(self.bias_constraint)
}
base_config = super(Conv, self).get_config()
return dict(list(base_config.items()) + list(config.items()))
def _compute_causal_padding(self, inputs):
"""Calculates padding for 'causal' option for 1-d conv layers."""
left_pad = self.dilation_rate[0] * (self.kernel_size[0] - 1)
if getattr(inputs.shape, 'ndims', None) is None:
batch_rank = 1
else:
batch_rank = len(inputs.shape) - 2
if self.data_format == 'channels_last':
causal_padding = [[0, 0]] * batch_rank + [[left_pad, 0], [0, 0]]
else:
causal_padding = [[0, 0]] * batch_rank + [[0, 0], [left_pad, 0]]
return causal_padding
def _get_channel_axis(self):
if self.data_format == 'channels_first':
return -1 - self.rank
else:
return -1
def _get_input_channel(self, input_shape):
channel_axis = self._get_channel_axis()
if input_shape.dims[channel_axis].value is None:
raise ValueError('The channel dimension of the inputs '
'should be defined. Found `None`.')
return int(input_shape[channel_axis])
def _get_padding_op(self):
if self.padding == 'causal':
op_padding = 'valid'
else:
op_padding = self.padding
if not isinstance(op_padding, (list, tuple)):
op_padding = op_padding.upper()
return op_padding
음…….
이것만 보고는 알기 힘들다. PyTorch의 소스도 가져와보겠다.
PyTorch
# PyTorch Conv2d
# https://github.com/pytorch/pytorch/blob/master/torch/nn/modules/conv.py
class Conv2d(_ConvNd):
def __init__(
self,
in_channels: int,
out_channels: int,
kernel_size: _size_2_t,
stride: _size_2_t = 1,
padding: _size_2_t = 0,
dilation: _size_2_t = 1,
groups: int = 1,
bias: bool = True,
padding_mode: str = 'zeros' # TODO: refine this type
):
kernel_size = _pair(kernel_size)
stride = _pair(stride)
padding = _pair(padding)
dilation = _pair(dilation)
super(Conv2d, self).__init__(
in_channels, out_channels, kernel_size, stride, padding, dilation,
False, _pair(0), groups, bias, padding_mode)
def _conv_forward(self, input, weight):
if self.padding_mode != 'zeros':
return F.conv2d(F.pad(input, self._reversed_padding_repeated_twice, mode=self.padding_mode),
weight, self.bias, self.stride,
_pair(0), self.dilation, self.groups)
return F.conv2d(input, weight, self.bias, self.stride,
self.padding, self.dilation, self.groups)
def forward(self, input: Tensor) -> Tensor:
return self._conv_forward(input, self.weight)
PyTorch의 경우도 Conv2d 클래스는 ConvNd를 상속받고 있으므로 ConvNd 클래스를 보도록 하겠다.
# PyTorch Conv2d
# https://github.com/pytorch/pytorch/blob/master/torch/nn/modules/conv.py
class _ConvNd(Module):
__constants__ = ['stride', 'padding', 'dilation', 'groups',
'padding_mode', 'output_padding', 'in_channels',
'out_channels', 'kernel_size']
__annotations__ = {'bias': Optional[torch.Tensor]}
_in_channels: int
out_channels: int
kernel_size: Tuple[int, ...]
stride: Tuple[int, ...]
padding: Tuple[int, ...]
dilation: Tuple[int, ...]
transposed: bool
output_padding: Tuple[int, ...]
groups: int
padding_mode: str
weight: Tensor
bias: Optional[Tensor]
def __init__(self,
in_channels: int,
out_channels: int,
kernel_size: _size_1_t,
stride: _size_1_t,
padding: _size_1_t,
dilation: _size_1_t,
transposed: bool,
output_padding: _size_1_t,
groups: int,
bias: Optional[Tensor],
padding_mode: str) -> None:
super(_ConvNd, self).__init__()
if in_channels % groups != 0:
raise ValueError('in_channels must be divisible by groups')
if out_channels % groups != 0:
raise ValueError('out_channels must be divisible by groups')
valid_padding_modes = {'zeros', 'reflect', 'replicate', 'circular'}
if padding_mode not in valid_padding_modes:
raise ValueError("padding_mode must be one of {}, but got padding_mode='{}'".format(
valid_padding_modes, padding_mode))
self.in_channels = in_channels
self.out_channels = out_channels
self.kernel_size = kernel_size
self.stride = stride
self.padding = padding
self.dilation = dilation
self.transposed = transposed
self.output_padding = output_padding
self.groups = groups
self.padding_mode = padding_mode
# `_reversed_padding_repeated_twice` is the padding to be passed to
# `F.pad` if needed (e.g., for non-zero padding types that are
# implemented as two ops: padding + conv). `F.pad` accepts paddings in
# reverse order than the dimension.
self._reversed_padding_repeated_twice = _reverse_repeat_tuple(self.padding, 2)
if transposed:
self.weight = Parameter(torch.Tensor(
in_channels, out_channels // groups, *kernel_size))
else:
self.weight = Parameter(torch.Tensor(
out_channels, in_channels // groups, *kernel_size))
if bias:
self.bias = Parameter(torch.Tensor(out_channels))
else:
self.register_parameter('bias', None)
self.reset_parameters()
def reset_parameters(self) -> None:
init.kaiming_uniform_(self.weight, a=math.sqrt(5))
if self.bias is not None:
fan_in, _ = init._calculate_fan_in_and_fan_out(self.weight)
bound = 1 / math.sqrt(fan_in)
init.uniform_(self.bias, -bound, bound)
def extra_repr(self):
s = ('{in_channels}, {out_channels}, kernel_size={kernel_size}'
', stride={stride}')
if self.padding != (0,) * len(self.padding):
s += ', padding={padding}'
if self.dilation != (1,) * len(self.dilation):
s += ', dilation={dilation}'
if self.output_padding != (0,) * len(self.output_padding):
s += ', output_padding={output_padding}'
if self.groups != 1:
s += ', groups={groups}'
if self.bias is None:
s += ', bias=False'
if self.padding_mode != 'zeros':
s += ', padding_mode={padding_mode}'
return s.format(**self.__dict__)
def __setstate__(self, state):
super(_ConvNd, self).__setstate__(state)
if not hasattr(self, 'padding_mode'):
self.padding_mode = 'zeros'
음……
솔직히 아직도 직관적이지 않다.
아니, 사실 굉장히 직관적이고,정말 천재들은, 구글과 페이스북의 유수의 인재들은 이렇게 코딩하는구나… 하며 감탄까지 자아내게 만드는 소스 코드이다.
하지만 필자 같은 중생이 한 눈에 보고 내부 구조가 파악될 코드는 결단코 아니다.차라리 내부 구조를 더 깊게 알고 싶다면 C++이나 C언어로 짜여진 코드를 보는 게 나을 것 같다.
Conv2D C/C++ Source Code & Further Specific Structure
아래 소스 코드는 순차적으로 C, C++로 포팅된 소스코드이다.
# Conv2D in C Language
void conv(double filter[FILTERSIZE][FILTERSIZE], double e[][INPUTSIZE], double convout[][INPUTSIZE]) {
int i = 0, j = 0;
int startpoint = FILTERSIZE / 2;
for(i = startpoint; i < INPUTSIZE - startpoint; ++i) {
for(j = startpoint; j < INPUTSIZE - startpoint; ++j) {
convout[i][j] = calcconv(filter, e, i, j);
}
}
}
double calcconv(double filter[][FILTERSIZE], double e[][INPUTSIZE], int i, int j) {
int m, n;
double sum = 0;
for(m = 0; m < FILTERSIZE; ++m) {
for(n = 0; n < FILTERSIZE; ++n) {
sum += e[i - FILTERSIZE / 2 + m][j - FILTERSIZE / 2 + n] * filter[m][n];
}
}
return sum;
}
C 소스 코드는 아래 깃헙 링크에서 볼 수 있다.
underflow101/MLDL
Machine Learning & Deep Learning Source Code. Contribute to underflow101/MLDL development by creating an account on GitHub.
github.com
# Conv2D in C++ Language
#include "Convolution2D.h"
namespace Convolution2D
{
int getDesiredOutputRes(const int& width_in, const int& width_filter, const int& stride, const int& padding)
{
return (width_in - width_filter + 2 * padding) / stride + 1;
}
// input_image = prev_laver, output_image = next_layer
void forward(const ConvFilter2D& filter, const ConvImage2D& input_image, ConvImage2D& output_image)
{
for (int j_in_base = -filter.j_padding_, j_out = 0; j_in_base < input_image.height_ + filter.j_padding_ - filter.j_res_ + 1; j_in_base += filter.j_stride_, j_out++)
for (int i_in_base = -filter.i_padding_, i_out = 0; i_in_base < input_image.width_ + filter.i_padding_ - filter.i_res_ + 1; i_in_base += filter.i_stride_, i_out++)
{
T sum = (T)0;
for (int j_f = 0, j_in = j_in_base; j_f < filter.j_res_; j_f++, j_in++)
for (int i_f = 0, i_in = i_in_base; i_f < filter.i_res_; i_f++, i_in++)
{
if (input_image.isValid(i_in, j_in) == true)
sum += input_image.getValue(i_in, j_in) * filter.getWeight(i_f, j_f);
// else // zero padding
}
sum += filter.getBias();
output_image.getValue(i_out, j_out) = sum;
}
}
// intput_grad = gradient of prev layer, output_grad = gradient of next layer
void backward(const ConvFilter2D& filter, const ConvImage2D& output_grad, ConvImage2D& input_grad)
{
for (int i = 0; i < input_grad.width_ * input_grad.height_; i++)
input_grad.values_[i] = 0.0;
for (int j_in_base = -filter.j_padding_, j_out = 0; j_in_base < input_grad.height_ + filter.j_padding_ - filter.j_res_ + 1; j_in_base += filter.j_stride_, j_out++)
for (int i_in_base = -filter.i_padding_, i_out = 0; i_in_base < input_grad.width_ + filter.i_padding_ - filter.i_res_ + 1; i_in_base += filter.i_stride_, i_out++)
{
for (int j_f = 0, j_in = j_in_base; j_f < filter.j_res_; j_f++, j_in++)
for (int i_f = 0, i_in = i_in_base; i_f < filter.i_res_; i_f++, i_in++)
{
if (input_grad.isValid(i_in, j_in) == true)
input_grad.getValue(i_in, j_in) += filter.getWeight(i_f, j_f) * output_grad.getValue(i_out, j_out);
// else // zero padding
}
}
}
void updateWeights(const T& eta_, const T& alpha_, const ConvImage2D& output_grad, const ConvImage2D& input_act, ConvFilter2D& filter)
{
VectorND<T> filter_weights_temp(filter.i_res_ * filter.j_res_ + 1);
filter_weights_temp.copyPartial(filter.weights_, 0, 0, filter.weights_.num_dimension_);
filter.weights_.assignAllValues((T)0);
for (int j_in_base = -filter.j_padding_, j_out = 0; j_in_base < input_act.height_ + filter.j_padding_ - filter.j_res_ + 1; j_in_base += filter.j_stride_, j_out++)
{
for (int i_in_base = -filter.i_padding_, i_out = 0; i_in_base < input_act.width_ + filter.i_padding_ - filter.i_res_ + 1; i_in_base += filter.i_stride_, i_out++)
{
for (int j_f = 0, j_in = j_in_base; j_f < filter.j_res_; j_f++, j_in++)
for (int i_f = 0, i_in = i_in_base; i_f < filter.i_res_; i_f++, i_in++)
{
if (input_act.isValid(i_in, j_in) == true)
{
filter.getWeight(i_f, j_f) += eta_ * output_grad.getValue(i_out, j_out) * input_act.getValue(i_in, j_in);
}
// else // zero padding
}
// update bias
filter.getBias() += eta_ * output_grad.getValue(i_out, j_out); // bias = 1 is assumed
}
}
// momentum
for (int f = 0; f < filter.weights_.num_dimension_; f++)
{
filter.weights_.values_[f] += alpha_ * filter.delta_weights_.values_[f];
filter.delta_weights_.values_[f] = filter.weights_.values_[f];
filter.weights_.values_[f] += filter_weights_temp.values_[f];
}
}
}
C++ 소스 코드는 아래 깃헙 링크에서 볼 수 있다. (라이센스는 "Do whatever you want License"라 마음대로 퍼왔다.)
jmhong-simulation/2016FallCSE2022
Contribute to jmhong-simulation/2016FallCSE2022 development by creating an account on GitHub.
github.com
역시, 로우레벨 언어(?)로 보니 확연히 그 구조가 눈에 띈다.
Conv2D는 4중첩 for문으로,
무려 O(N^4)의 시간복잡도를 지닌다.
허허.
그렇다면 어째서 위처럼 4중첩 for문이 되었을까?
Conv2D는 이미지 텐서 인풋의 x축과 y축의 시작, 끝지점까지 하나하나의 픽셀을 검사하며 각각의 픽셀에 컨벌루션곱을 실행하며 (이때 필터를 적용) 2차원 배열 convout[i][j]에 이 정보를 저장하여 텐서 형식으로 아웃풋을 낸다. (이때 이미지 텐서 인풋의 x축과 y축을 모두 검사하기 때문에 2중첩 for문이 된다.)
각각에 픽셀에 대응하여, 컨벌루션곱을 진행하는데, 이 곱셈은 아래의 수학적 식을 갖는다.
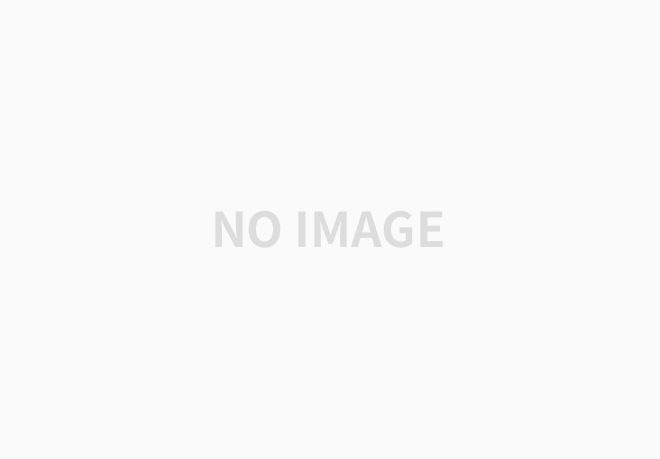
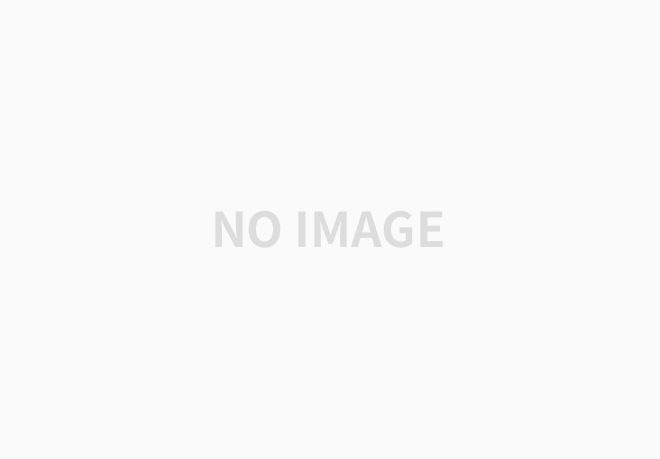
만약 위 식처럼, h[t], x[t]라는 시간에 대한 이산신호 두 가지가 있다고 가정할 때,
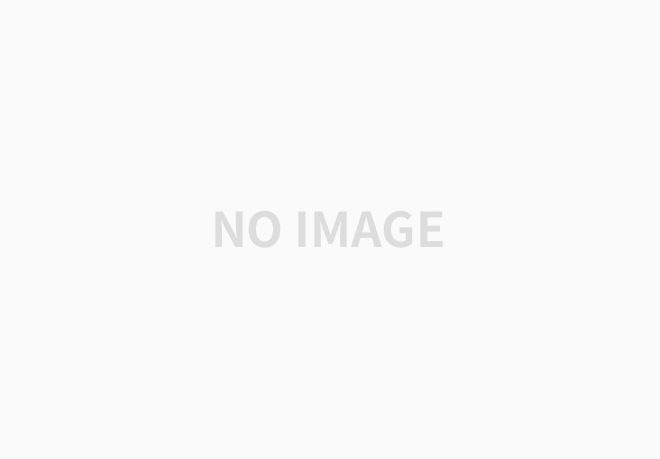
두 이산신호의 컨벌루션 곱(Convolution Product/합성곱)은 위 일반식으로 표현할 수 있다.그리고 위 일반식을 해당 h[t]와 x[t]에 적용한 경우,
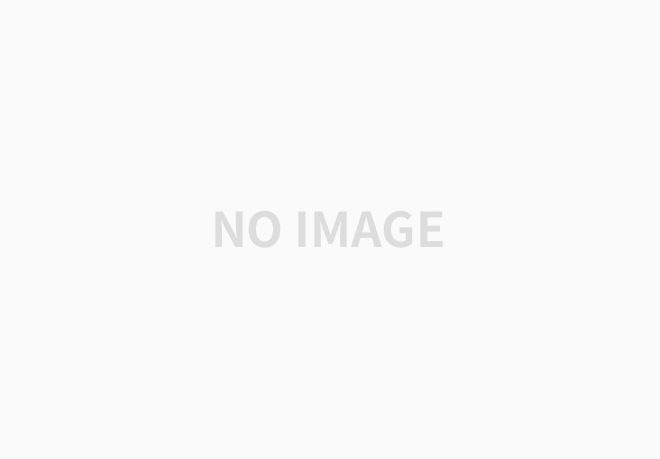
위와 같은 식들이 탄생하게 된다.
그렇기 때문에 각각에 픽셀에 대해 2중첩 for문이 새롭게 적용되어 각각 컨벌루션 곱을 진행하게 되고, 그 결과값을 반환하게 된다.
그렇기 때문에 최종적으로는 4중첩 for문이 되며 시간복잡도는 O(n^4)가 된다.
추가적으로, Conv2D의 파라메터 개수는 K2CM개가 되는데,
이때, K는 Filter의 크기이며 Input의 채널의 수는 C이며, Output의 채널의 수는 M이다.
즉, 하나의 Filter가 가지는 크기는 K2C이며, 이를 M개의 채널로 만들어주어야 하기 때문에 K2CM이 되는 것이다.
Computational Cost, 즉 연산량은 Input의 크기가 H × W라고 할 때, Output 또한 H × W가 되기 때문에,
하나의 Filter당 총 H × W번 연산을 해야 하나의 Output Channel이 만들어지게 된다.따라서 총 연산량은 K2CMHW가 된다.
이 포스팅이 도움이 됐길 바란다.
Tensorflow 2.X의 Conv2D 내부 구조가 궁금했던,PyTorch의 Conv2d 내부 구조가 궁금했던,Conv2D의 시간복잡도, 명시적으로 눈에 띄는 구조 및 원리가 궁금했던,
이 포스팅에서 해결이 됐길 바라며,또 다음 포스팅에서 이어가도록 하겠다.
'AI & Big Data > AI' 카테고리의 다른 글
[AI 이론] Layer, 레이어의 종류와 역할, 그리고 그 이론 - 5 (DepthwiseConv, PointwiseConv, Depthwise Separable Conv) (0) | 2020.07.09 |
---|---|
[AI 이론] Layer, 레이어의 종류와 역할, 그리고 그 이론 - 4 (Pooling Layer) (0) | 2020.07.08 |
[AI 이론] Layer, 레이어의 종류와 역할, 그리고 그 이론 - 2 (BatchNormalization) (2) | 2020.07.07 |
[AI 이론] Layer, 레이어의 종류와 역할, 그리고 그 이론 - 1 (Conv2D) (2) | 2020.07.06 |
[Keras] SqueezeNet Model (CNN) 이란? - 2 (실전편, 소스코드 첨부) (0) | 2020.02.13 |